Natural Language Generation (NLG) | Auto-Updating Copy | Automated Texts + Product Descriptions | GPT + RPA + DeepL
GPT-4: What You Need to Know And What's Different From GPT-3 And ChatGPT
Reading Time 16 mins | March 13, 2023 | Written by: Anne Geyer
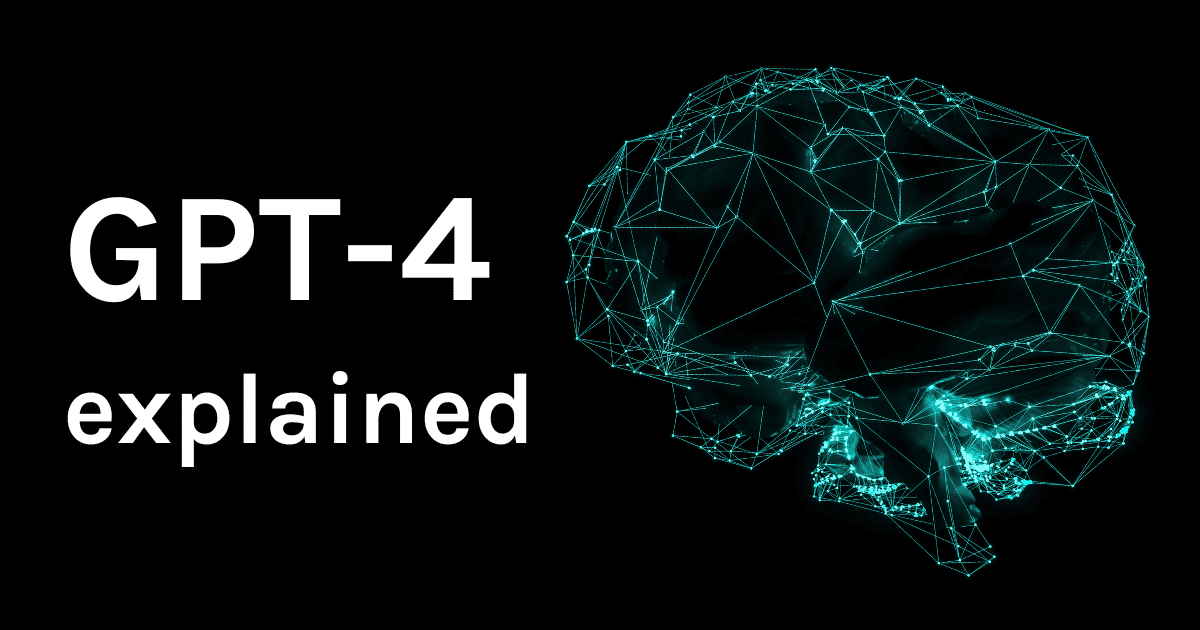
As ChatGPT is still all the rage and has seen one million registrations in the first couple of days after its release, OpenAI has released their latest language model GPT-4, the long awaited successor of GPT-3. GPT-4 is a further development of GPT-3, or GPT3.5, bringing with it a range of new capabilities and enhancements that make it more powerful than its GPT-predecessors – at least according to OpenAI.
But what is GPT-4 all about and what can it do and where are its limitations? Read all about what we know about GPT-4 (so far) in the following.
The contents of this article are:
- What is GPT And What Can It Be Used For?
- GPT-3 vs. data-to-text – What’s the difference?
- What Is GPT-4?
- What is The Difference Between GPT-4 And Its Predecessor GPT-3?
- What Does GPT-4 Look Like?
- Scope of Application for GPT-4
- What Is ChatGPT
- What Is The Use of ChatGPT?
- The Limitations Of ChatGPT
- What is the future of GPT?
1. What is GPT And What Can It Be Used For?
GPT stands for Generative Pre-trained Transformer and is a model that uses deep learning to produce human-like language. The NLP (natural language processing) architecture was developed by OpenAI, a research lab founded by Elon Musk and Sam Altman in 2015.
GPT uses a large corpus of data to generate human-like language representations. It is a language model that learns from existing text and can provide different ways to end a sentence. It has been trained with hundreds of billions of words, representing a significant portion of the internet - including the entire corpus of the English Wikipedia, countless books, and a dizzying number of webpages.
GPT can be used for various tasks and many practical applications such as summarization, question answering, translation, market analysis, and much more.
The latest model of the GPT series is GPT-3. But there is another big language model that generates texts automatically: data-to-text. But how do these two models differ and in which cases are they applicable?
2. GPT-3 vs. Data-to-Text – What’s the Difference?
Both GPT-3 and data-to-text are NLG technologies. NLG means "Natural Language Generation" and refers to the automated generation of natural language text. At first glance, they may seem quite similar, but they work very differently. Some of these differences are listed here:
Differentiators | Data-to-Text | GPT-3 |
Text generation by | based on structured data (attributes available in, e.g., tables, like product features from a PIM system or match results of a soccer game) | learns from existing text (trained with hundreds of billions of words from, e.g. Wikipedia, books, and numerous web pages) |
Control over content | user has control over text result at all times | user has no control over generated content |
Text quality | text consistency, meaningfulness, and quality | texts need to be fact checked; might give wrong or improper information |
Scalability | texts are customizable and scalable | generate individual texts |
Languages | multilingual content creation in up to 110 languages | multilingual content creation on a per-language basis possible |
Usage | For creating large amounts of text based on structured data sets with variable details | To create a basic text; can simplify writing process |
Because of these differing qualities, GPT-3 and data-to-text are suitable for different applications.
Data-to-text is used in e-commerce, the financial and pharmaceutical sector, in media and publishing. GPT-3 can be helpful for brainstorming and in finding inspiration, for example, if the user is suffering from writer’s block. To use GPT-3 in chatbots to answer recurring customer queries is quite useful as well, as having humans generate the text output is inefficient and impractical. |
It is a fact that GPT tools can be a good complement for drafting texts or as inspiration for writing. With GPT-powered writing integrated into AX Semantics' data-to-text software, there are hardly any limits to more creativity with increased efficiency and time savings at the same time.
Learn more about GPT-3 vs. Data-to-text: What’s the Right Content Generation Technology for Your Business? or download our one-pager on the difference between GPT and data-to-text free of charge:
3. What Is GPT-4?
GPT-4 is the latest version of the GPT models developed by OpenAI. As with any of the GPTs, GPT-4 is being trained on a massive amount of data and able to generate human-like text for multiple tasks. It is able to produce high-quality content, including blog articles, reports, and news articles.
It was released on March 14, 2023 and is OpenAI's most advanced system and is considered an improvement over previous models in the series in terms of performance on various metrics. OpenAI says that GPT-4 is "10 times more advanced than its predecessor, GPT-3.5. This enhancement enables the model to better understand context and distinguish nuances, resulting in more accurate and coherent responses."
4. What Is The Difference Between GPT-4 And Its Predecessor GPT-3?
As GPT-3 has already left quite an impression in its capabilities after its release in 2020, expectations for GPT-4 were high. Anyways, in brief, the improvements of GPT-4 in comparison to GPT-3 and ChatGPT are it’s ability to process more complex tasks with improved accuracy, as OpenAI stated. This allows for a wider range of applications.
The biggest difference between GPT-3 and GPT-4 is shown in the number of parameters it has been trained with. GPT-3 has been trained with 175 billion parameters, making it the largest language model ever created up to date. In comparison, GPT-4 is likely to be trained with 100 trillion parameters. At least that’s what Andrew Feldman, CEO of Cerebras said he learned in a conversation with OpenAI. Some argue that this amount of parameters brings the language model closer to the workings of the human brain in regards to language and logic.
If you would like to try out the differences in results between GPT3.5 and GPT-4 for yourself as part of a Data2text application, you can choose between the two models in our platform.
Size doesn’t matter – GPT-4 won’t be bigger than GPT-3
However, in its goal to mimic the human language, GPT-4 has have a huge advantage over GPT-3 for its training on so many parameters and huge data input. It is a big step forward in the GPT models capability of analyzing text input and processing inquiries. Because of its comprehensive training, GPT-4 also gives a lot more choices of sentence continuations as well as voices and styles.
In conclusion, this means that GPT-4 will think even more human-like than any other GPT model so far. Quite astounding, as in a Q&A at the AC10 online meetup in 2021, OpenAI’s CEO Sam Altman said that GPT-4 would not be bigger than GPT-3 and wouldn’t exceed GPT-3 in size. Altman explained that OpenAI’s goal is not to build a massive language learning model, but to focus on improving their GPT models’ performances.
As we explained above, data-to-text uses structured data provided by the user as the basis for content generation. This means that the user has more control over text design and text quality. So if you are considering using content automation, you should take a close look at which of the two technologies is right for your requirements. In this video, AX Semantics CEO Saim Alkan explains the difference between content results from data-to-text tools like AX Semantics and those from ChatGPT:
5. What Does GPT-4 Look Like?
GPT-4 can be trained on any language dataset. Next to data, OpenAI has also focused on the improvement of algorithms, alignment and parameterization. As a GPT model, it has an improved transformer architecture for a better understanding of relationships between words in text.
What GPT-4 looks like:
1. Alignment
Alignment is posing a big challenge for OpenAI. As they aim for their language models to be able to interact with and understand the intentions of users, they also need the AI to align with our (moral) values. This is still a challenge for the GPT models and also discussed as regards ChatGPT. According to OpenAI, to improve in terms of alignment, GPT-4 has been trained with "more human feedback, including feedback submitted by ChatGPT users" and they "applied lessons from real-world use" of their previous models" to improve GPT-4’s safety research and monitoring system. OpenAI also announced that ChatGPT will be "updating and improving GPT-4" regularly. Still, the alignment problem remains. It is also unclear whether OpenAI filtered the human feedback according to any parameters or if it was used entirely unfiltered.
2. Parameterization
As mentioned above, according to Andrew Feldman, GPT-4 was trained with more parameters than its predecessors, so it relies on more data to learn from. According to OpenAI, the improved accuracy was achieved through additional training of the AI, in which humans evaluated the fact accuracy. However, OpenAI claims that the output answers of GPT-4 are "40% more likely to produce factual responses than GPT-3.5."
3. Multimodality
Fans of automated image creation or even automated video creation, might be disappointed by GPT-4. But as Altman had already pointed out preemptively, GPT-4 only produces texts and, therefore, focuses solely on language generation. But GPT-4 is a multimodal model anyway as it can take images as input.
4. Transformer Architecture
As any GPT model, GPT-4 uses transformer architecture. A transformer architecture allows for a better understanding of relationships between words in text. Hence, it allows for improved accuracy in language understanding tasks.
5. Accuracy
According to Open AI, GPT-4 has significantly improved in accuracy. The company claims that GPT-4 is 40% more accurate than GPT-3.5, but it remains unclear how they measure the accuracy improvement. However, with an improved accuracy, GPT-4 is able to carry out various tasks, such as text generation and summarization. As GPT-4 also has more advanced capabilities for natural language understanding, it will consequently have an enhanced understanding of context or of a given task and complete it more accurately than GPT-3. GPT-4 is also designed to handle larger amounts of data and more sophisticated tasks than GPT-3. In some tests that were actually designed for humans, GPT-4 has shown to be able to pass more exams than ChatGPT.
6. Overall Usability
GPT-4 has larger context windows. That means that users can generate longer texts and larger input than before. According to OpenAI, GPT-4 is “capable of handling over 25,000 words of text, allowing for use cases like long form content creation, extended conversations, and document search and analysis”. OpenAI also claims that GPT-4 has a better performance on different languages and has an improved integrated steerability, which means that users are able to change style, tone etc. more easily.
6. Scope Of Application For GPT-4
As an improvement of GPT-3, GPT-4 has a wider range of applications and can be used in many different areas such as natural language processing (NLP), machine translation, speech synthesis and understanding. GPT-4 can also be used for tasks that require deep understanding of text, such as summarizing or comprehension. GPT-4's advanced algorithms allow it to perform these tasks more effectively than GPT-3 and ChatGPT.
In particular, GPT-4, as a language model, will find application in professions and businesses that need or are linked to content and content creation. In marketing and sales, GPT-4 can, for example, be used to outline and write (ad) campaigns. This also goes for writers and content creators, as they may use GPT-4 even more as a source of inspiration and help to write and generate content with GPT-4. However, as it is not always a reliable source, fact checking is always advisable.
Furthermore, with GPT-4 the content output is not scalable. Therefore, it is not suitable for cases where a lot of content is needed and where it is imperative that the given information is true and appropriate.
With a data-to-text software like AX Semantics, texts are configured only once initially in the tool, the content output scales rapidly. For e-commerce companies, data-to-text is profitable because they can, for example, very quickly generate high-quality product descriptions for hundreds or thousands of products – even in different languages. This can save time and money, as well as increase SEO visibility and conversion rates on product pages.
7. What Is ChatGPT?
GPT-3 was used as a basis to build the NLP model ChatGPT, which is capable of understanding and generating human-like conversations in real time.
ChatGPT is an AI-chatbot. It’s designed to generate natural and human-like conversation responses in real-time, and is able to do so in many different languages – one at a time. ChatGPT stems from a model in the GPT-3.5 series and is described by OpenAI as a “fine-tuned” version of it.
It was designed to give users quick, precise, and helpful answers to their questions. So its main purpose is to respond to text questions in an informative or entertaining way. ChatGPT has been trained until early 2022. That means, it can generate texts about events and developments up until this point in time.
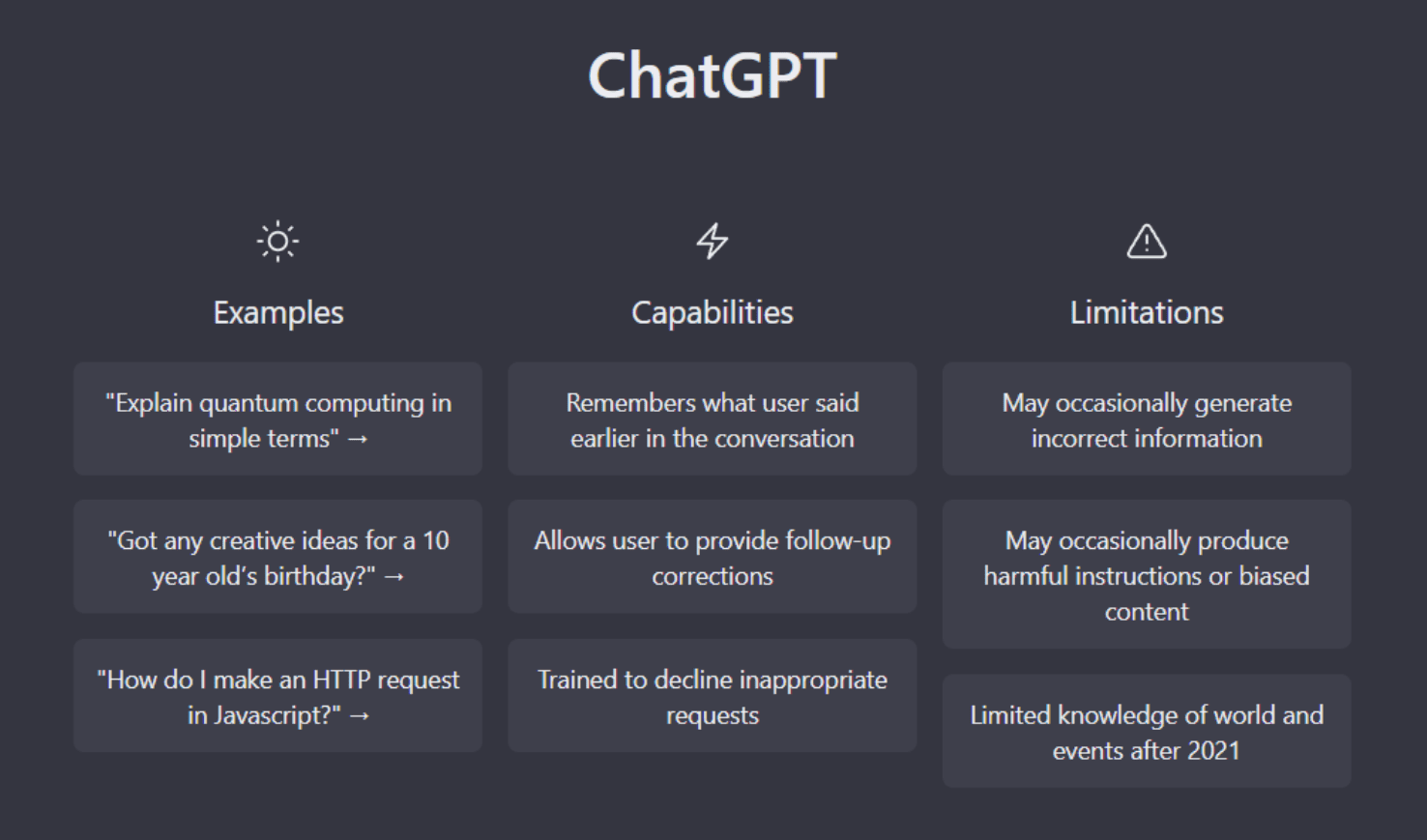
7.1 What Is The Use Of ChatGPT?
ChatGPT can be used for various conversational tasks, ranging from customer service to online dialogue recommendation. It can be used to build virtual assistants and chatbots that can generate natural conversations with humans.
It also understands and writes code in different programming languages. Therefore, the chatbot can be used for debugging codes, and can explain it as well as help to improve it. In a more general sense, ChatGPT is great at explaining complex things and issues in simple and easy to understand words.
7.2 The Limitations Of ChatGPT
As the hype over ChatGPT has been enormous, it also has its weaknesses. For example, the language model can give plausible-sounding, but incorrect or nonsensical answers. While ChatGPT is trained on a vast amount of text, it still lacks the common sense knowledge that humans possess. This means that it may sometimes provide inaccurate or incomplete responses to questions or tasks that require contextual understanding or background knowledge.Therefore, it is strongly recommended to fact check ChatGPTs answers. And it's not likely that this issue will be fixed any time soon. As OpenAI writes on their website:
“Fixing this issue is challenging, as: (1) during RL training, there’s currently no source of truth; (2) training the model to be more cautious causes it to decline questions that it can answer correctly; and (3) supervised training misleads the model because the ideal answer depends on what the model knows, rather than what the human demonstrator knows.”
OpenAI
This is why, at least in some cases, answers can vary according to the way users formulate questions or their input phrases. This is also true, when the user gives an ambiguous inquiry. Instead of asking for clarification, ChatGPT will guess the users' intent behind the question and answer accordingly. This is very well shown in this example:
THE 🔑 misunderstanding of chatGPT: when it tells us that the very much alive Noam Chomsky died in 2020, it’s not “drawing on sources.”
— Gary Marcus (@GaryMarcus) December 8, 2022
It’s drawing on bits of text that were never previously connected & confabulating a relationship between those bits—without checking the data pic.twitter.com/dweDO5bavB
Also, ChatGPT does not have the ability to understand emotional context, such as sarcasm, irony, or humor. This means that it may sometimes provide responses that are tone-deaf or inappropriate, particularly when dealing with sensitive or emotional topics. This also goes for the generation of creative or original responses. While ChatGPT is capable of generating text, it is limited by the data it has been trained on, and may not be able to produce truly original or creative responses to questions or prompts. Furthermore, the texts can reflect biases and inaccuracies from the texts the software has been trained with and contain responses that are offensive, inappropriate, or reflect societal biases.
For these reasons, it is important to use ChatGPT with caution and recognize its limitations when relying on its responses. There are also alternatives for ChatGPT that you can try.
8. What Is The Future Of GPT?
GPT-4 is a big step for natural language learning models. Future GPT models will likely be even more powerful, create more human-like texts, and sooner or later, will be able to produce multimodal content, as it will combine text, image, and video creation. As time goes by, the GPT models will get even better and have much larger capacity as well as greater accuracy. Tackling more and more complex tasks, such as natural language generation, machine translation, and question answering.
To become even more similar in its workings to the human brain, the AI’s role model, sparsity is another issue that will need to be addressed in the future. However, sparsity might not be so easy to achieve and the alignment problem might not be so easy to overcome.
While GPT-4 will be more powerful than GPT-3, ChatGPT is currently a good option for those looking to experiment with GPT-3 technology, and while away the time until GPT-4 will be released.
In this video, OpenAI’s CEO Sam Altman talks about the future of AI and the alignment problem in more detail:
FAQ
Natural Language Generation (NLG) refers to the automated generation of natural language by a machine. As a part of computational linguistics, the generation of content is a special form of artificial intelligence. Natural language generation is used in many sectors and for many purposes, such as e-commerce, financial services, and pharmacy sector. It is seen to be most effective to automate repetitive and time-intensive writing tasks like product descriptions, reports or personalized content. Learn more about Natural Language Generation (NLG).
Automated content generation with AX Semantics works with the help of Natural Language Generation (NLG) - a technology that generates high-quality and unique content on the basis of structured data that is
indistinguishable from manually written content. Text automation is used for generating product descriptions, category content, financial and sport reports or content for search engines websites. In a nutshell, it is used for all kinds of content that require large quantities and have a similar basic structure.
There are a multiple ways to use AI in content creation. You can use AI to help you come up with topics or ideas for articles, you can use it as a research tool to gather information, or it can help you to write and edit your content.
For instance, e-commerce businesses can use AI to create a chatbot that generates and displays customer service content. A chatbot is a computer program that can mimic human conversation. You can type in a topic or question, and the chatbot will respond with a list of relevant responses.
However, you can also use AI to create product descriptions, sales copies, CTAs, and more. It all depends on your business needs.
In short, data-to-text is used in e-commerce, the financial and pharmaceutical sector, in media, and publishing. GPT-3 can be helpful for brainstorming and finding inspiration, for example when the user is suffering from writer's block. Using GPT-3 in chatbots to answer recurring customer queries is also very useful, as having humans generate the text output is inefficient and impractical. For more in-depth information, visit our Data2Text Automation site