Natural Language Generation (NLG) | Automated Texts + Product Descriptions
AI Agents in Product Communication: Transforming Content Operations
Reading Time 8 mins | November 5, 2024 | Written by: Robert Weißgraeber
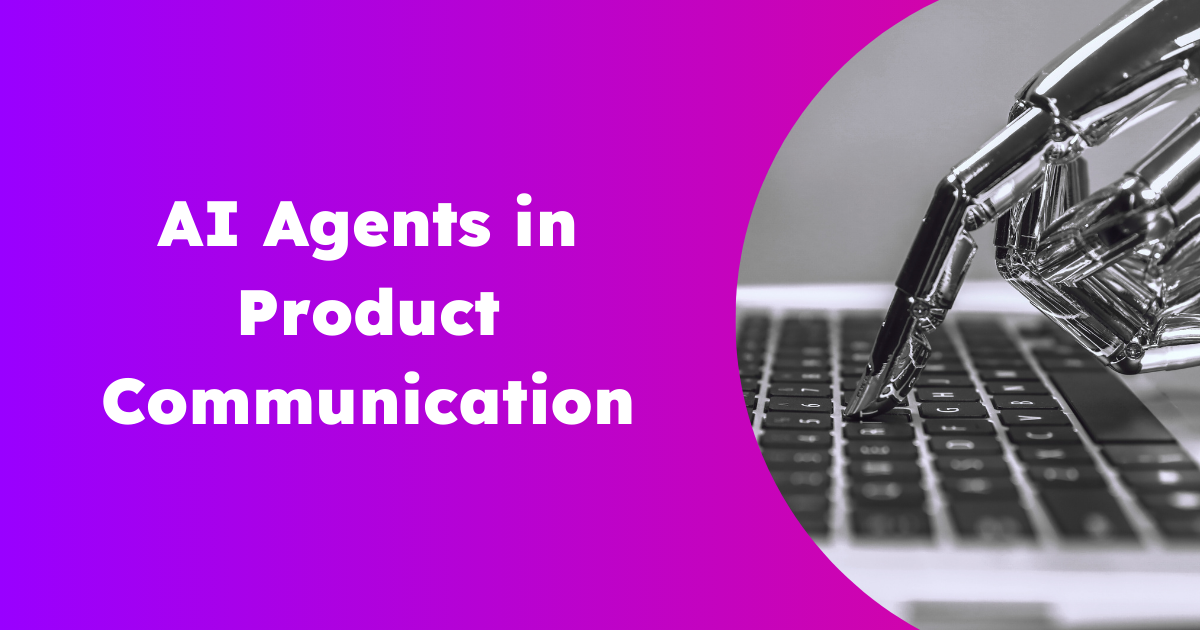
In today's content-driven digital environment, the integration of AI agents into content operations is fundamentally transforming how businesses create, optimize, and manage their digital communications. From e-commerce to content marketing, AI agents for Natural Language Generation (NLG) are at the forefront of scaling content production, reducing costs, and enhancing quality. This article provides an in-depth exploration of AI agents in content generation, covering key aspects of data-driven content operations, content automation, multi-language capabilities, and best practices for successful e-commerce content. We will address the principles of effective content operations, the critical role of automation, and emerging trends in AI-powered content creation.
Content
1. Understanding AI Agents in Content Generation
2. Key Components of AI Agents for Content Generation
3. Automation as a Key Principle
4. Success Factors for Good Content in E-Retail and E-Commerce
5. Multi-Language Capabilities and Associated Costs
6. Use Cases and Applications of AI Agents in this context
7. Challenges and Considerations in Using AI Agents
8. AI Agents and the Future of Content Operations
9. Building LLM-Based AI Agents: A Practical Guide
10. How AI Agents Support Innovation and Experimentation
1. Understanding AI Agents in Content Generation
AI agents are advanced software entities capable of performing complex tasks autonomously, enhancing the efficiency of content creation. Unlike traditional automation, which follows static rules, AI agents can combine multiple methods of content creation, analyze impacts, and allow operators to adjust their output accordingly. In content generation, AI agents employ machine learning and NLP technologies to produce automation pipelines for human-like narratives for various kinds of product content, including product descriptions.
In the content ecosystem, AI agents have emerged as game-changers by automating mundane writing tasks, analyzing user preferences, and creating personalized content. Companies like AX Semantics have leveraged these capabilities to develop data-driven content operations, integrating AI agents to streamline content workflows.
2. Key Components of AI Agents for Content Generation
The key components of data-driven AI agents for content generation include data analysis, planning mechanisms, and tools that allow user interaction to facilitate a human-machine collaboration, enabling the user to add fine-grained domain expertise. The core or "brain" of the AI agent, usually based on a Large Language Model (LLM), such as GPT-4 or Mistral, takes the user-defined objectives and derives the processes needed to generate content effectively. These LLMs are trained on vast corpora of data, which allow them to provide accurate and context-aware content outputs.
Advanced AI agent pipelines also add Quality Assurance components to allow corporations specific control over language, brand voice, compliance, etc., further enhancing autonomous operations.
Memory systems, including both short-term and long-term memory, help AI agents retain context over multiple interactions, making content more coherent and user-specific. Tools like retrieval-augmented generation (RAG) allow AI agents to access external databases, providing up-to-date information for content that requires factual accuracy.
3. Automation as a Key Principle for Content Operations
Automation is fundamental to optimizing content operations, especially when considering the challenges of scalability and cost-efficiency. For e-commerce and digital marketing teams, managing large-scale content output manually can be time-consuming and resource-intensive. AI agents facilitate content automation by handling repetitive tasks, allowing content strategists and writers to focus on creativity, ideation, and impact.
Modern Content Operation platforms demonstrates the power of AI Agents in automation: reducing bottlenecks and ensuring consistency in content production. Automation also supports quality assurance, reducing errors and maintaining brand voice, even across high-volume content campaigns.
4. Success Factors for Good Content in E-Retail and E-Commerce
Effective e-commerce content should be clear, engaging, and optimized for conversion. AI agents contribute to success in e-commerce by generating content that is customer-centric and tailored to drive engagement. Product descriptions, for instance, need to be both informative and compelling, which AI agents can achieve by analyzing market trends and consumer preferences.
Another critical factor is SEO optimization. From a technical SEO perspective, AI agents can incorporate trending keywords, generate meta-descriptions, and optimize headings, ensuring that product pages rank higher on search engines. This leads to increased visibility and improved sales, making AI a valuable asset in content marketing for e-retail.
From a content SEO perspective, using AI agents in a data-to-text fashion to set up autonomous processes allows for immediate updates when data changes. This capability enables content to include more detailed information, such as classification of attributes (e.g., the size of a TV set in relation to the entire market of available sizes) or seasonality, making the content significantly more valuable to the reader and enhancing content quality signals. This content also drives visibility for specific products, enhancing AIEO (Artificial Intelligence Experience Optimization).
5. Multi-Language Capabilities and Associated Costs
One of the significant challenges in content operations is the ability to cater to a global audience. AI agents equipped with multilingual capabilities offer a cost-effective solution for content translation and localization. Instead of traditional translation methods, which can be costly and time-consuming, AI agents use neural machine translation to create content in multiple languages while preserving tone and intent.
The ability to generate multi-language content efficiently helps companies enter new markets with minimal additional costs. AI agents can also adapt their outputs based on cultural nuances, which is crucial for connecting with diverse audiences in a meaningful way.
6. Use Cases and Applications of AI Agents in Content Generation
AI agents are versatile, capable of generating many content types across different contexts. For product-related content, this includes:
-
Product Descriptions: These agents can produce SEO-optimized descriptions that are both informative and persuasive.
-
Category Pages: AI agents can create engaging category pages that summarize the product offerings effectively, helping potential customers navigate through available options and boosting overall page engagement.
-
Filter Pages: AI agents can generate dynamic content for filter pages, which helps customers find specific products based on selected criteria like color, size, or price. This makes the shopping experience more personalized and efficient.
-
Collection Pages: AI agents can produce well-curated content for collection pages, grouping products based on themes or occasions, enhancing user experience and encouraging multiple purchases.
-
Transactional Content: Personalized email, such as basket-recovery emails generated by AI agents, can significantly boost open and click-through rates by providing tailored messaging that resonates with customers' past interactions and preferences.
By integrating AI agents into their content strategies, companies can accelerate production, maintain high quality, and reduce costs associated with traditional writing processes, which includes maintaining the content over time, not just production efforts.
7. Challenges and Considerations in Using AI Agents
Despite their many benefits, AI agents come with challenges, including issues related to bias, content originality, and ethical concerns. AI agents are trained on large datasets that may contain inherent biases, leading to skewed perspectives if not adequately managed. Regular monitoring and the inclusion of diverse training datasets can mitigate these risks.
Another consideration is the potential lack of originality. AI agents are adept at reusing patterns from training data, which may lead to less creative or overly formulaic outputs. Human intervention remains crucial for adding a creative touch that makes the content resonate with its intended audience.
For product copy, "grounding" the output in data is key: By linking the content to product data, the facts in the content are connected to hard data, reducing the risk of hallucinations and increasing the specificity of the content.
8. AI Agents and the Future of Content Operations
The role of AI agents in content generation will continue to expand, with advancements in language models and machine learning techniques driving this evolution. Future trends include the development of multimodal AI agents capable of integrating text, audio, and visual content seamlessly. Such advancements could revolutionize content operations, particularly in sectors like education and entertainment.
Moreover, we will likely see AI agents taking on more responsibilities, such as content planning and performance analysis, offering end-to-end content solutions that cover ideation to execution. This shift will further reduce the burden on human creators, freeing them to focus on strategy and innovation.
For this to work in a business context, automated content production needs impact information. This can be derived by combining measurement capabilities, like on-site tracking for traffic and conversion, and by integrating with Business Intelligence (BI) systems to track cost-side factors like return rates or call center costs for product support.
9. Building LLM-Based AI Agents: A Practical Guide
Creating a content-generating AI agent involves several steps, starting with selecting an automation platform that allows access to various content generation methods and LLMs. Businesses need to identify their content needs and use that to inform their choice of AI model. Training the model with domain-specific data helps ensure that the generated content aligns with brand expectations, and adding specific content expectation briefings provides guidance on brand/product specifics.
Next, integrating the AI agent into existing content workflows is essential for seamless operation. This can involve linking the AI model to a content management system (CMS) to automatically publish content or to an analytics tool to monitor content performance.
10. How AI Agents Support Innovation and Experimentation
AI agents empower creative professionals by taking over repetitive tasks, thereby giving them the freedom to innovate. By handling the initial drafting stages, AI agents allow content creators to experiment with different formats, topics, and ideas. The collaboration between AI and human creativity results in more dynamic and diverse content outputs. This is further enhanced by allowing editors to make choices between different tools for different challenges, avoiding the need for a "one-size-fits-all LLM" that generates everything from product titles to personalized emails. Breaking the content production task down into smaller areas allows for flexible choices, and testing enables data-driven decisions.
Conclusion
AI agents are rapidly becoming indispensable tools for content operations. They enhance scalability, reduce production costs, and improve overall content quality—all while empowering human writers to contribute domain knowledge, focus on strategic and creative aspects of content production. As AI technology continues to evolve, its role in content generation will only grow, making it an essential component of modern content strategies in an increasingly digital world.
Key Factor is always the integration with tools to measure and prove success – making business impact a first-class citizen for making (data-driven) decisions in the editorial team.
Robert Weißgraeber
Robert Weissgraeber is Co-CEO and CTO at AX Semantics, where he heads up product development and engineering and is a managing director. Robert is an in-demand speaker and an author on topics including agile software development and natural language generation (NLG) technologies, a member of the Forbes Technology Council. He was previously Chief Product Officer at aexea and studied Chemistry at the Johannes Gutenberg University and did a research stint at Cornell University.